Overview
On Tuesday 7th May 2013, the Department of Mathematical Sciences at Durham University will host a Workshop with topic "Geometrical Structures in Statistics".
The draft programme for the meeting is shown below; the venue will be the Department of Mathematical Sciences, Durham University
(marked 15 on the map).
The workshop will be linked to a meeting of the North-Eastern local group of the Royal Statistical Society (RSS).
(Of course, the local RSS session at 16:30 can still be attended without attending the full workshop.)
All talks will take place in Room CM 221 unless stated otherwise. Registration, coffee, and buffet lunch will be in CM 103.
Scope
Shape analysis; Spatial modelling; Principal graphs, trees, and manifolds; Evolutionary trees; and any other topics which relate to the overall theme of the workshop.
|
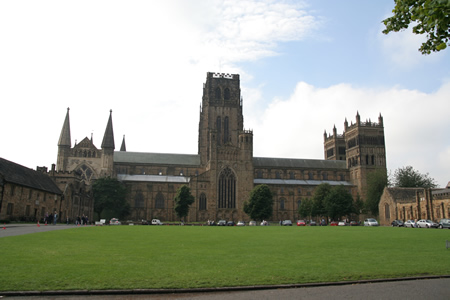

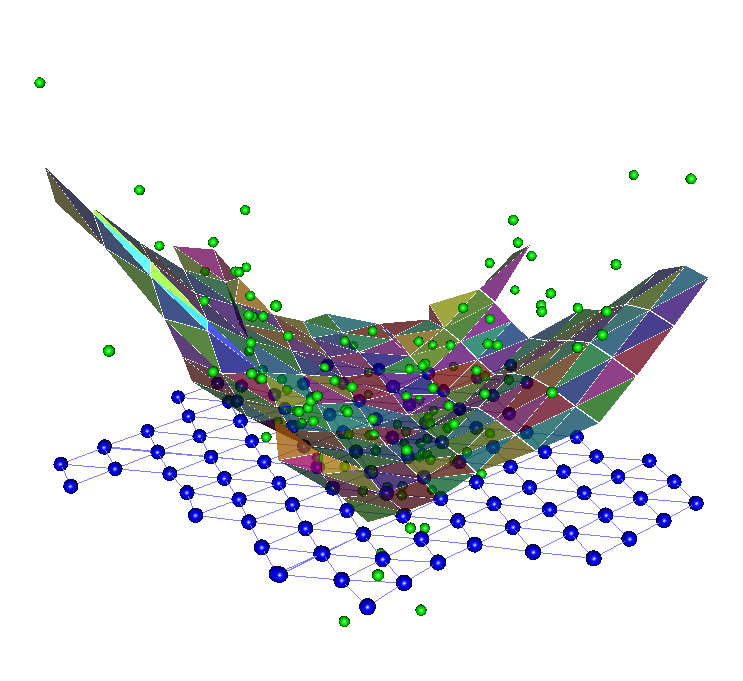
 |
|
Programme
10:00-10:15 | REGISTRATION (CM 103)
|
10:15-11:00 | KEYNOTE I (E 005) Alexander Gorban, University of Leicester
Growing Robust Principal Graphs for Data Approximation
(joint work with E.M. Mirkes and A. Zinovyev)
Revealing of hidden geometry and topology in data sets with presence of noise is a very challenging task. Many branches of data analysis aim to solve them under some additional hypotheses that simplify the problem. For example, if we assume that the data form several groups of similar objects with significant dissimilarity between groups then we approach the clustering problem, whereas if we assume that the data are situated along a low-dimensional manifold then we need the manifold learning approach. However, the verification of these assumptions may be more complicated than the solutions of the problems. A universal technology for revealing of the hidden structure is very desirable. An answer to this challenge cannot be simple because it must potentially cover the majority of the situations. This should be a sort of language with good expressiveness. Each data set may be considered as a hidden text in this language and our goal is to reveal this text.
The main message of our talk is a presentation of a good candidate for this language and the revealing technic. For this purpose, we used the bunch of ideas and approaches developed by various authors in data mining and neural networks:
- The oldest of them is the idea of self-consistency introduces by H. Steinhaus in 1957 (k-means) and then recognized as a very general and productive idea that can be used for construction of many principal objects like principal manifolds and principal graphs (Husty at al, 1984). It is an intrinsic part of the self-organizing maps (SOM) and many data approximation approaches as well.
- The application of quadratic elastic energy functionals is a basic idea of the spline approximation and is used by us for construction of principal manifolds in 1996, in the elastic maps technology.
- The growth technic was implemented in the growing SOMs and in the growing elastic maps methods.
- The classical idea of graph grammars was transformed by us into the topological grammars approach for data analysis (2007).
- In 2008, we proposed the pluriharmonic embedments of graphs into data space as the ideal approximators.
- The idea that makes all this bunch of approaches much more efficient is the robust growth.
For organization of robust grows, we use the truncated energy functionals. In the splitting algorithms of optimization they
also produce the systems of linear equations, and make the construction of the approximators much more stable in presence of noise and outliers.
We describe the new algorithms and present the systematic comparison of different algorithms on the benchmarks. The new technology gives the optimal combination of the global thinking and local view.
|
11:00-11:20 | COFFEE (CM 103)
|
11:20-11:40 | Thomai Tsiftsi, Durham University (CM 221)
Shape
modelling for the statistical analysis of sandbodies
TBA
| 11:40-12:00 | Daniel Bonetti, USP, Sao Carlos, Brazil (CM 221)
Estimation of Distribution Algorithms for Protein Structure Prediction
Proteins are present in almost all processes of life and are essential to keep organisms working. Knowing the configuration of a protein that causes a disease it is possible to develop medicines. During decades, scientists have been developed medicines using experimental methods. However, such methods require a lot of money and time. For this reason, computational methods have also been developed to try finding protein configurations, knowing as Protein Structure Prediction (PSP) problem. These methods are classified in knowledge-base and template-free. First, uses knowledge from proteins already determined by experimental methods. Other, as used in this work, uses the amino acid sequence and force field potential to look for the smallest energy configuration. In order to obtain the smallest energy configuration we use an evolutionary algorithm called Estimation of Distribution Algorithm (EDA). EDAs are capable to estimate values of variables at each generation of the evolutionary process basing
only on the values of a relevant set of solutions. We know that the distribution of variables of PSP problem is multi modal and multivariate. Thus, basing on kernel method, we developed two version of our algorithm: Univariate and Multivariate EDA. We noticed that Univariate EDA runs faster than the Multivariate. Besides, considering that it is an optimization problem of minimization, the Univariate was able to get the lowest value, but the Multivariate was able to find the best configuration with a slightly worst energy. In this talk, I will explain the PSP problem, a short explanation of Evolutionary Algorithms and how it is possible to sample new values from variables with multi modal for univariate and multivariate case using kernel estimation.
| 12:00-12:20 | Ayodeji Akinduko, University of Leicester (CM 221)
Multiscale Principal Component Analysis
Principal Component Analysis (PCA) is an important tool in exploratory data analysis which is useful in revealing interesting structures in multivariate data. The conventional approach to PCA leads to a deterministic solution which favours the structures with large variances. This is sensitive to outliers and could obfuscate interesting underlying structures especially for data with multiscale structures. One of the equivalent definitions of PCA is that it seeks the projection that maximizes the square sum of the pairwise distances of data and this definition opens up some flexibility and control in the analysis of principal components which can be useful in enhancing the performance of PCA. In this paper we introduce scales into the analysis of the principal components by maximizing only the sum of pairwise distances within a chosen scale, thereby influencing the resulting principal components. This method was tested using various scales on artificial distribution of data with multiscale
structures and the method was able to reveal the different structures of the data. It can be concluded that Multiscale PCA is useful for revealing the structures in data with multiscale structure which conventional approach to PCA could not reveal.
| 12:20-13:10 | BUFFET LUNCH (CM 103)
|
13:10-13:40 | Ian Jermyn, Durham University (CM 221)
Random field shape models of networks and multiple objects
-- This talk had to be cancelled!
|
13:40-14:10 | Tom Nye, University of Newcastle (CM 221)
Geometric statistics for evolutionary trees
Most conventional statistical methods assume that the data lie in a Euclidean vector space. In contrast, evolutionary trees and other tree-like objects live in geometrical spaces which lack much of the structure required for typical statistical modelling, and this makes analysing sets of trees particularly challenging. In this talk I give a brief introduction to the geometry of evolutionary tree space and describe how sample means and variances can be computed. I will also describe methods for inferring principal geodesics. Principal geodesics reveal and quantify the principal sources of variation in a collection of trees, and they can be visualized as animations of smoothly changing alternative evolutionary trees.
|
14:10-14:30 | SHORT BREAK
|
14:30-15:00 | Hujun Yin, The University of Manchester (CM 221)
Manifold Representations of Facial Images and Their Variations
The curse of dimensionality has prompted intensive search for effective methods of mapping high dimensional data, esp. images. Dimensionality reduction and subspace/manifold learning have been studied extensively and widely applied to feature extraction and pattern representation in face recognition or related image and vision applications. Many nonlinear methods such as the kernel PCA, local linear embedding, Laplacian eigenmap and self-organising networks have been proposed recently for dealing with these complex image data sets. Complexity, however, increases dramatically when further variations such as pose, lighting and expression are considered. A single manifold become inadequate to capture the structures of such data and few existing methods have excelled in practical applications under unconstrained environments. This talk will first summarise the existing methods for representing facial images. Then various test results on several benchmark data sets will be shown and
illustrated on the capabilities of these methods. Finally an open question is posted on using multiple manifolds in representing the variations.
|
15:00-15:30 | Peter Jupp, University of St Andrews (CM 221)
Statistics of Orthogonal Axial Frames
An orthogonal axial frame is a set of orthonormal vectors which are known only up to sign. Such frames arise in seismology, crystallography and as principal axes of multivariate data sets. This talk will outline work with Richard Arnold (Wellington) on the development of methods of analysing data that are orthogonal axial frames.
|
15:30-15:50 | Uwe Kruger, Sultan Qaboos University, Muscat, Oman (CM 221) (joint work with J. Einbeck and Z. Kalantan)
Identification of models for linear and nonlinear non-causal data structures
Part I, Part II
| 16:00-16:30 | COFFEE (CM 211)
|
16:30-17:20 | KEYNOTE II (Local RSS session, CM 221)
Robert Aykroyd, University of Leeds, UK
Geometric models for object tracking with electrical tomography data
Industrial processes require constant monitoring to improve efficiency and to give early warning of potential hazards. Electrical tomography provides a cheap and non-invasive approach allowing the interior of pipes, reaction vessels etc. to be monitored using only voltage measurements from the exterior. In a standard analysis the electrically conductivity distribution within the region of interest is discretized leading to a very large number of unknown parameters. Stable solution of this ill-posed inverse problem is not possible unless additional assumptions can be made—in the Bayesian setting this involves defining suitable prior distributions. The estimated conductivity distribution can then be used to visualize the process. For automatic monitoring and control an image representation is not ideal. Instead it may be more useful to parameterize in terms of physical parameters which can be used directly. To motivate the proposed approach the separation of water and oil in a hydrocyclone will be
considered. The model is now of a homogeneous object within a contrasting homogeneous background. There is still scope for the inclusion of prior information on boundary smoothness and likely conductivity values, for example. Simulations will be described, including of the monitoring and control of the water-oil hydrocyclone example.
|
17:20-17:30 | DISCUSSION AND CLOSE (CM 221)
|
General Details
Registration
Please register by Monday 15th of April 2013 if you would like to attend (please specify any dietary requirements).
For registrations received by the deadline, the registration fee will be £10 (which includes buffet lunch and coffee), with postgraduate students attending (and lunching) free.
For late registrations (after 15th of April 2013) the registration fees increases to £20 (£10 for postgraduate students).
The registration fee is payable on the day.
Link to registration form
Abstract Submission
If you are interested in giving a talk or presenting
a poster, please submit talk title and abstract by Monday, 8th of April 2013 to one of the two e-mail addresses given in the Contacts section.
Postgraduate students are particularly welcome to attend and present their work.
In case of more submissions than available slots, a selection will be made by the local organizing committee.
Abstracts may be accepted before the official submission deadline. In any case, notifications will be sent at the latest by 12th of April.
Accommodation
If you are planning an overnight stay, then information
on suitable accommodation (en-suite rooms, B&B, in Durham colleges) is available here. All colleges
offer suitable accommodation, if you wish a recommendation then we would suggest Collingwood College.
For those arriving the day before the workshop, there are plans to go for an informal get-together on the evening of the 6th of
May (at participants' own costs) in a local pub. Please let us know if you would like to join.
Travel
The Department is easily accessible by bus, taxi or foot from the
railway station. More information about travelling to Durham can be found here and here. A useful map
can be found here, where the Department of Mathematical Sciences is marked (15).
Car parking at the Department of Mathematical Sciences is in extremely limited supply. For those arriving by car, you would need to let us know at
registration (at least 3 weeks in advance) if you need a visitor parking permit --- it will not
be possible to obtain a day-permit at the entrance barriers.
A preferable alternative would be to use the Durham Park and Ride at the nearby Howlands Park site which offers a large supply of secure parking.
Contact
In case of any questions, or to submit your abstract, please contact Jochen Einbeck (0191 3343125) or
Ian Jermyn (0191 3343080).
Local Organizing Committee: Jochen Einbeck, Ian Jermyn, Jonathan Cumming, Michael Racovitan
Notes
We provide two travel bursaries over 80£ each, for postgraduate students. Interested students need to register by the deadline, and provide the organizers with a short CV.
This event is supported by the Department of Mathematical Sciences at Durham University,
the North-Eastern local group of the Royal Statistical Society (RSS), and Santander Universities UK.
|